It is not a secret that combining web design with machine learning has opened up many new options. This article will discuss how machine learning is added to web design and what tools and trends can be utilized.
Machine learning, which is part of artificial intelligence, has made a significant impact on web design. It helps do repetitive tasks automatically and adjusts content to fit each person, making websites better for everyone.
Understanding how machine learning works is critical to seeing how it changes web design. Machine learning makes websites work better for users by using patterns in data.
So, let’s move forward and understand the role of machine learning in modern web design in detail.
The Role of Machine Learning in Web Design
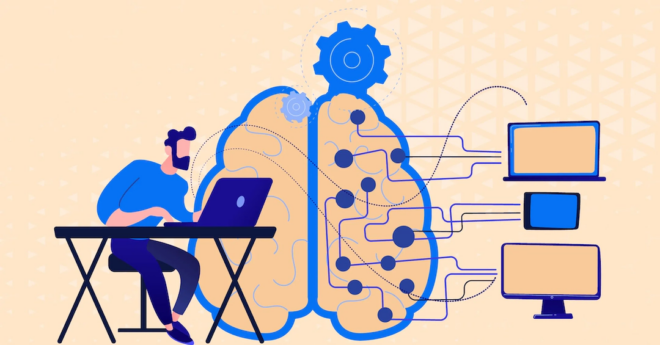
- What is machine learning?
Machine learning happens when a computer uses data and results to make a program that can be used in regular programming. Traditional programming is when a computer uses data and a program to make an outcome.
Even though machine learning is a part of artificial intelligence, they are not the same. In machine learning, machines learn to improve at tasks without exact programming, while artificial intelligence aims to make machines think and decide things like humans.
- How does machine learning improve web design?
Machine learning helps web developers make websites better for users by customizing them using visitors’ information and actions. For instance, machine learning models can suggest items or content that fit users’ past actions and likes. Many streaming services already use this. That cool song you found in your “recommended” list? Machine learning likely put it there.
- Examples of Machine Learning Applications in Web Development
The first example concerns content generation and improvement. Machine learning helps improve content by suggesting ways to enhance SEO, checking how users engage, and making short summaries.
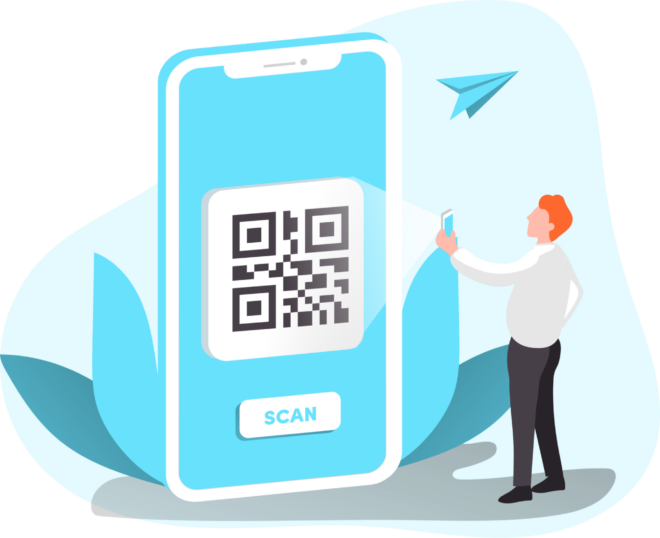
The second example is about customizing QR codes. With innovative computer methods, websites can create a QR code for a URL. It will change based on how users act, where they are, or what they like. This makes QR codes more personal and helps track users’ actions.
The last thing machine learning can be helpful for is predicting how users act. Websites use special computer programs called machine learning models to guess or estimate how users might behave. For example, they might suppose what users will buy or click on next.
Automating Tasks in Web Design Using Machine Learning
Machine learning makes designing websites easier by doing repetitive jobs, letting designers use their time better. It can suggest colors, fonts, and layouts based on users’ liking and current design trends. This helps designers start quickly and build from there.
Machine learning helps with coding and making websites, too. It looks at existing code, learns from it, and suggests or even creates parts of new code. This helps coders write better code and find mistakes faster, improving the final website.
- Popular tools and practices
There are tools and tech that use machine learning for automating tasks. Some have AI helpers for design or code that work with popular coding programs. TensorFlow and PyTorch are frameworks for making custom machine-learning tools for web design jobs. Another use is chat APIs for faster responses and enhanced customer service. Several chats use machine learning for intelligent features like natural language processing (NLP) to understand and respond to user messages. There are many chat APIs, that can be helpful for a web designer. Among them are Twilio, Sendbird, and a great Sendbird alternative Sceyt.
Personalizing Content with Machine Learning
Making content personal is essential for web design. It helps websites connect better with users. Machine learning is a big help in doing this.
- Importance of Personalization in Web Design:
Personalizing content matters a lot in web design. When websites show things that users like or are interested in, it keeps them engaged and interested in coming back.
- How Machine Learning Enables Content Personalization
Machine learning helps in making content personal. It looks at what users do on a website, like what they click on or read, and then suggests similar things. This makes the website more tailored to each user’s preferences.
- Implementing Personalization Algorithms in Web Design:
Special computer programs (algorithms) are used to make websites personalized. These programs analyze user behavior and suggest content that users might like. Web designers use these algorithms to make the website more exciting and appealing.
- Tools for Personalization in Web Design
Various tools that use machine learning to personalize content and aid in web design are available. Tools like Canva provide easy-to-use interfaces for creating personalized graphics, allowing designers to tailor visual content based on user preferences and trends. Other machine learning-powered platforms, such as Adobe Sensei or Figma, offer features that analyze user data to suggest design elements, layouts, and styles that resonate with the target audience. Many Canva alternatives are there to help any web designer personalize their website’s visual content.
Conclusion
In the world of web design, machine learning is changing how designers make websites. It helps by doing repetitive tasks and making content personal for each person. This makes designing websites easier and makes users’ experiences better. Tools like Canva and Fotor use machine learning to suggest designs and create personalized content. As web design keeps growing, using machine learning tools will keep being important. They’ll help make sure websites are not just useful but also interesting and appealing to different people. Machine learning is shaping the future of how we experience the internet.